Abstract Background The assessment of hidradenitis suppurativa (HS) severity requires detailed, and error-prone lesion counts. This proof-of-concept study aimed to automatically classify HS disease severity using machine learning of clinical smartphone images. Methods 777 ambient-light and size-controlled images were used to build a class-balanced synthetic dataset (n = 7675). Convolutional neural networks (CNN) were used for automated severity classification (scale 0–3), and to assess disease-dynamics. International Hidradenitis Suppurativa Severity Score System (IHS4) served as reference. A U-NET algorithm was implemented for automated localization of diseased skin. Results CNNs were able to distinguish no/mild from moderate/severe disease with an overall prediction accuracy of 78% [receiver operating curve (AUC) 0.85]. Correct IHS4 classification was achieved with an overall accuracy of 72% (AUC 0.84–0.89). In addition, disease dynamics using IHS4 numerical values aligned with CNN outputs (NRMSE 0.262). The UNET algorithm localized lesions with a pixel accuracy of 88.1% and test loss of 0.42. Limitations Limitations in assessing tattooed and hairy skin. Limited number of patients with dark skin colour and Hurley I. Conclusion CNNs were able to distinguish no/mild from moderate/severe disease, classify disease severity over time, and automatically identify diseased skin areas and the skin phototype. This study breaks new grounds for fast, reliable, reproducible and easy-to-use HS severity assessments using clinical images.
Automated Classification of Hidradenitis Suppurativa Disease Severity by Convolutional Neural Network Analyses Using Calibrated Clinical Images
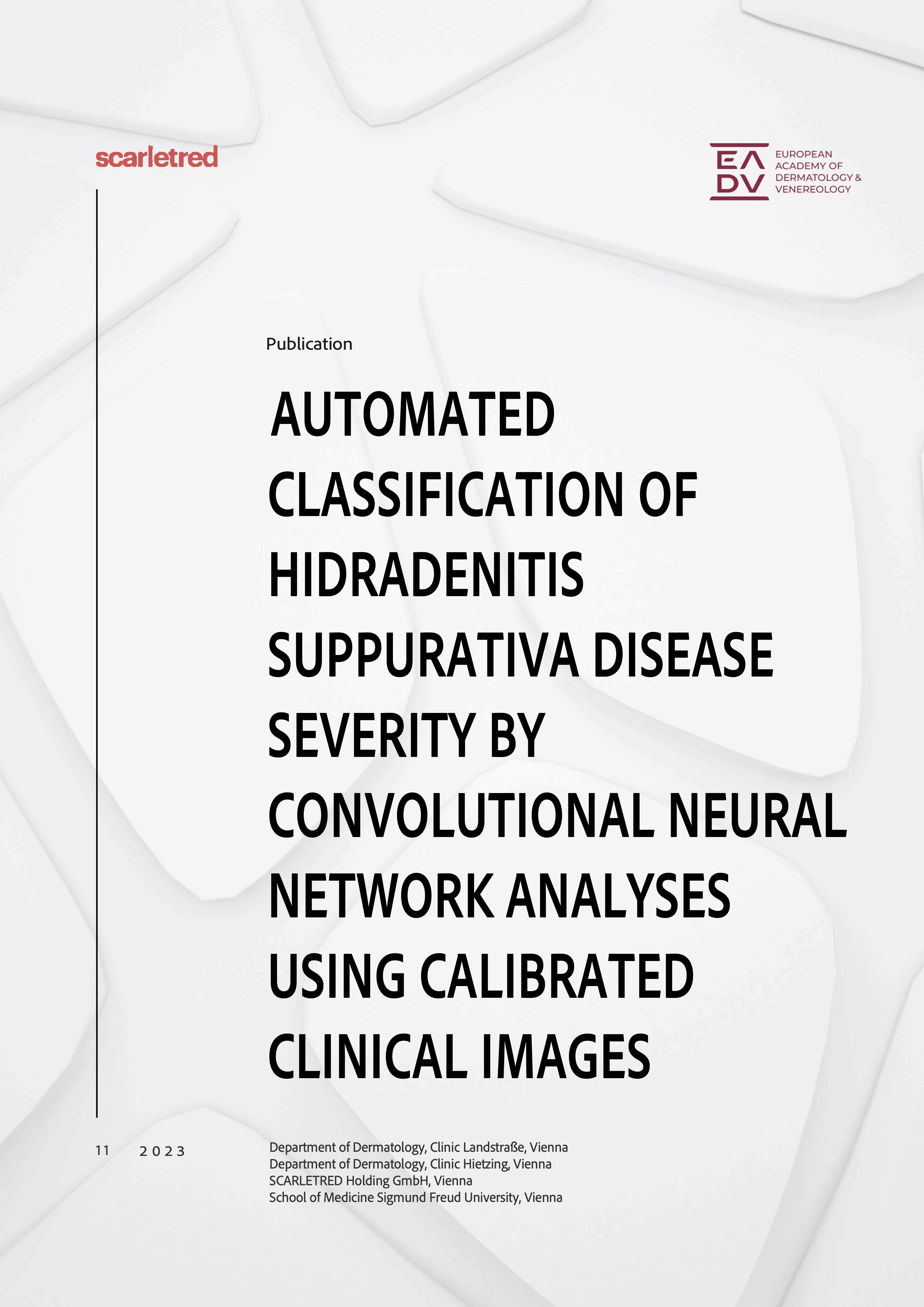
Download PDF
Let's get in touch!
Our state of the art digital platform is supplied via flexible SaaS agreement to leading corporates and newcomers worldwide. Connect with us to obtain more information in a personal demo, and to get a scalable solution fitting with your specific clinical study or piloting demands.